
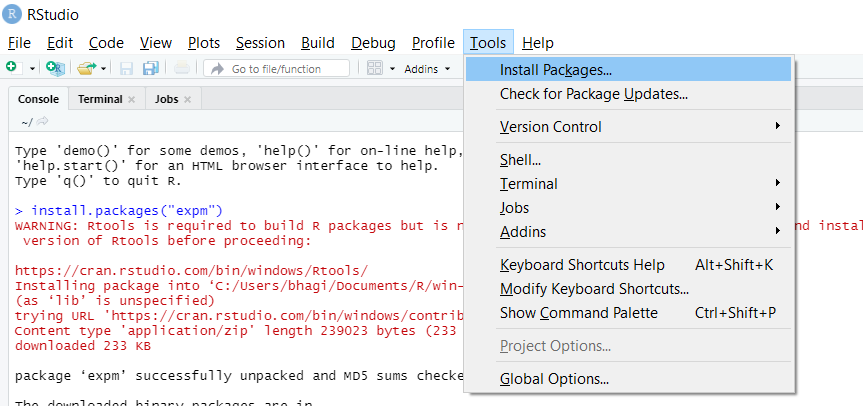
These packages are devtools and roxygen2. Now, let’s download two packages which are life savior in developing R packages. Maintaining the package version is a very important step and it has to be mentioned in the DESCRIPTION file correctly.ĩ. Since R packages are open-source tools, it is important to provide a suitable license for your package. Also, the next line must start with four spaces. The text under this title can be written in multiple lines and the text per line must be within some word limits. Steps in developing an R packageīe careful while writing the text under Description. Also, it discusses the possible warnings and corresponding solutions.
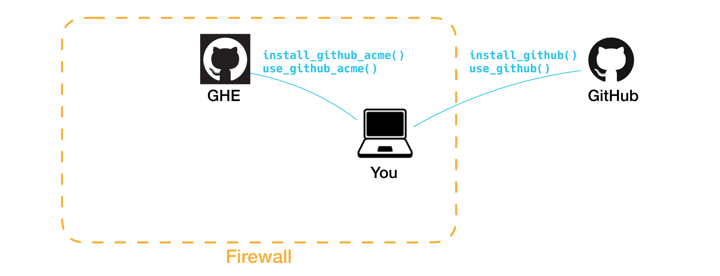
This post is to demonstrate the easiest steps to develop an R package with minimum efforts. Many researchers avoid developing the R package due to a lack of knowledge of possibilities and the tedious procedure to develop the R packages. Also, the R packages available in CRAN repository are well checked and can be uploaded to repository only after all warnings and errors are addressed by the package contributors, which ensures the error-free codes in the form of R package.
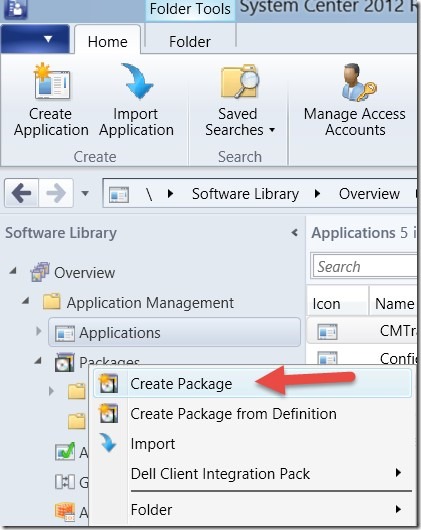
The R packages are documented well with description, vignette, sample examples, version controls, shareable code, integration with IDE (R Studio), license, and many more. On the contrary, the R packages are the complete environment for computational codes, which ensures all the covering over it, so that it can be used without any hassle. Besides, the version controls are the other serious issues. But, in alternative cases, if such codes are lacking with instruction manuals, documentation, and prerequisite sources or libraries, it is challenging to troubleshoot and reproduce the results.
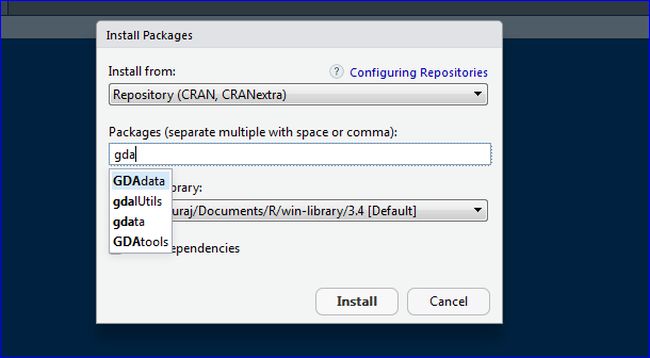
If such codes are documented and demonstrated well, the world can utilize it with boundless possibilities. Generally, GitHub is the preferred repository for many researchers to store and share their codes. Several researchers working in computational domain work on various interesting problem statements and end up with some meaningful code and analysis. The combination of such R packages makes the task of data analysis as easy as playing with some board games. There are several packages for data wrangling, data visualization, data predictions, datasets, optimizations, test benches, performance evaluation tools, and many more. The R packages are generally combinations of functions that are written in R and targeted for some data analysis functionalities. Nowadays, R packages are gaining huge popularity because of many reasons. The R packages are the open-source tools, generally used in analyzing or visualizing datasets.
